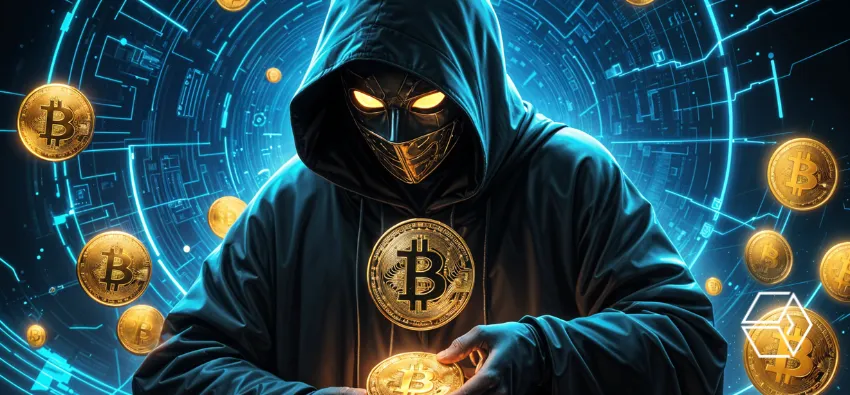
Elliptic conducted a study in collaboration with the MIT-IBM Watson AI Lab, which revealed that there is almost proof of fraud associated with Bitcoin transactions, including money laundering. This analysis followed a more extensive dataset on elliptic2, which included over 122,000 sub-graphs as well as a massive network of over 49 million unique clusters connected by 196 million identified transactions.
Another new dataset is this one, which is based on the previous version, the Elliptic Data Set (EDS), which was released at the end of 2019. The EDS used graph convolutional neural networks (GCNs) to detect financial irregularities.
According to Tom Robinson, the chief scientist and one of Elliptic’s founders, their anti-money laundering (AML) strategies differ from traditional methods. It uses machine learning techniques at a subgraph level, rather than just monitoring those coming from known illegal sources or detecting suspicious patterns with money laundering. A group of networked transactions forms this subgraph, and they can help uncover the correlation between certain activities and illicit proceeds.
The study applies complex subgraph classification techniques like GNN-Seg, Sub2Vec, and GLASS to analyze the complex interplay and dynamics in the cryptocurrency world. By this methodology, the fraudulent transactions that occur with crypto exchange accounts have been traceable. These suspect payments have been traced back to a variety of sources, including bitcoin mixers, Ponzi schemes in Panama, and a private Russian dark web community.
In his research, Robinson emphasized the significance of showing the “structure” of laundering graphs, which represent a certain pattern inside the network. During his study, he discovered several strategies for laundering money, including the so-called peeling chain, in which little amounts of cryptocurrency are gradually taken from the main pool and sent to different addresses. This method mirrors the classic financial practice known as “smurfing.”
The study’s results have demonstrated some great potential, as they recognize commonly used money laundering methods and predict future ones. This research aims to enhance the efficacy and accuracy of these techniques and expand their use to other blockchains, which significantly contributes to curbing these financial crimes anywhere in the world and ensuring the integrity of cryptocurrency transactions.